June 8, 2022
AI for achieving Critical View of Safety in Laparoscopic Cholecystectomy Videos
Laparoscopic cholecystectomies can be subject to complications such as bile duct injury, which can seriously harm the patient or even result in death. Computer-assisted interventions have the potential to prevent such complications by highlighting the critical structures (cystic duct and cystic artery) during surgery, helping the surgeon establish the Critical View of Safety and avoid structure misidentification. Currently, CVS achievement is manually assessed through recorded videos or doublet photography. The immediate goal was to establish an automated system to identify the CVS in LC videos.
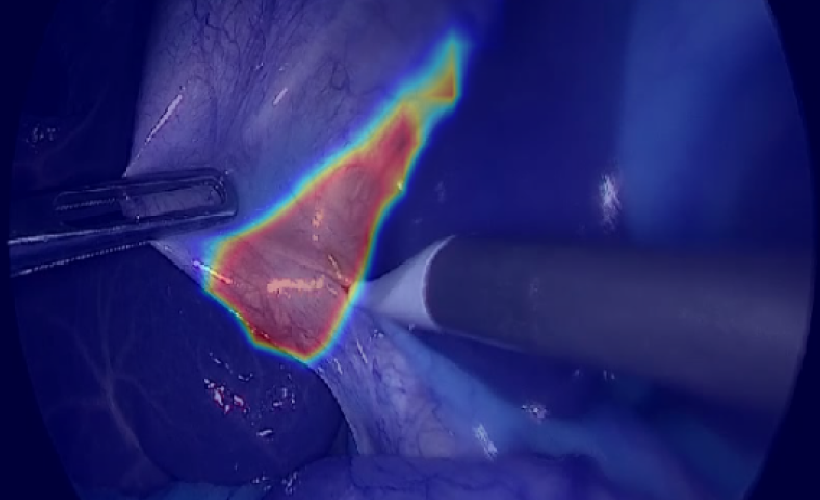
The first step was to select a two minute snippet from an LC video just before clipping of a structure, and then the extracted frames (one per second) were annotated by trained medical students based on the achievement of the three criteria for CVS: 1) seeing only two structures entering the gallbladder 2) exposing the inferior 1/3 of the cystic plate, and 3) clearing the hepatocystic triangle of fat and fibrous tissue. A deep learning model consisting of a Convolutional Neural Network (CNN) and a Recurrent Neural Network (RNN) was trained to detect the three CVS criteria. The model was evaluated using accuracy, precision (positive predicted value), sensitivity, and F1 metrics.
A total of 120 LC videos combing the cholec80 and EndoVis Datasets were used for training (80 videos) and validating (40 videos) the model. The accuracy and F1-score of our model were 0.98 and 0.81, respectively, with precision and sensitivity of 0.96 and 0.70. The total runtime for each frame was less than 0.01 seconds.
An AI model proved to be useful in detecting the CVS in LC videos. The proposed system could potentially be used online for real-time feedback generation and offline for objective performance assessment. Further studies are needed to assess the clinical impact of surgical data science solutions for safer laparoscopic cholecystectomy.
The development of a successful automated system to identify the CVS in LC videos will require a significant amount of quality data with pixel perfect image annotation. Medical Data Cloud is taking part in AI-powered technological progress by providing researchers with high-quality, custom datasets and highly accurate and affordable image annotation.
References:
1. Namazi, Babak, PhD “AI for Automated Detection of the Establishment of Critical View of Safety in Laparoscopic Cholecystectomy Videos”
2. Journal of the American College of Surgeons: October 2020 - Volume 231 - Issue 4 - p e48 DOI: 10.1016/j.jamcollsurg.2020.08.118
3. Pietro Mascagni “Formalizing video documentation of the Critical View of Safety in laparoscopic cholecystectomy: a step towards artificial intelligence assistance to improve surgical safety” DOI: 10.1007/s00464-019-07149-3